Why sample size is important in football analytics
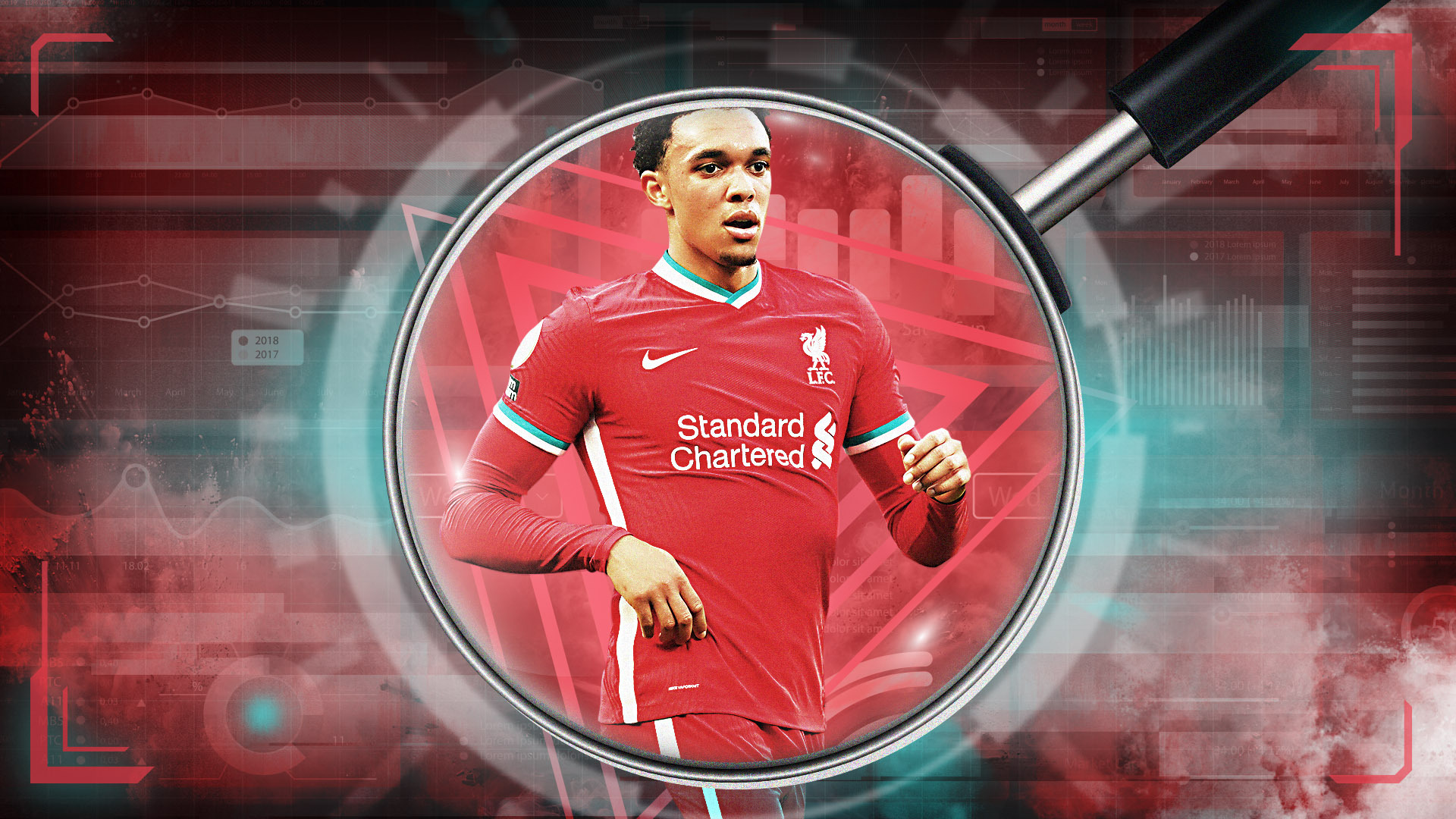
Has there ever been as much interest in football stats as there is now? Sky Sports, BT Sport and Match of the Day have all embraced the new offerings while banter accounts on social media now dabble in them, if only to antagonise a fanbase.
This is both a positive and a negative.
The more exposure people have to stats, the easier it is to introduce new ones into the mainstream. We have expected goals (xG) and it can’t be long before expected assists (xA) make their debut on TV.
It’s no longer an underground society and people don’t have to hide the fact they’re interested in this side of the game. However, with more stats now publicly available, people with no experience of using them are able to cherrypick and manipulate ones to suit narratives and agendas.
It’s why you often hear those working in this area talk about ‘sample size’. Analysing a player over a large period of time takes those hot streaks and poor patches into account. It helps paint a fairer picture of a player.
Think about it, your natural instinct in real life when seeing something amazing is “can you do it again?”. People usually want to make sure it isn’t a fluke.
I once knocked a tub of butter (Clover for those wondering – other butter brands are available) off the kitchen side and softened the fall with my foot before flicking it back up and catching it. For those few seconds, I was Ronaldinho. I’ve not tried to do it again so my record is still one for one and I can confidently say I am as good as the Brazilian maestro. You can’t prove otherwise.
It’s a small sample size, though. This is the sort of thing you look to avoid when analysing players. People sometimes think sample size is directly linked to minutes, but it can be the volume of actions, too.
Trent Alexander-Arnold was recently criticised for his performance against Southampton. He lost possession on 38 occasions and 25 of his 76 attempted passes didn’t find a team-mate. In isolation, both of those stats aren’t great.
But when you compare his numbers from the Southampton game over a large sample size – his Premier League stats since the start of 2018/19 – you get a better understanding of, well, everything.
Per Wyscout, Alexander-Arnold has averaged 63 passes per 90 and he completes 75%. Of those, 10 are usually deemed long passes and his accuracy with those is 51%. That’s a solid baseline to go off.
In his 77 minutes on the pitch at St Mary’s, the Liverpool right-back attempted 76 passes, of which 27 fell into the long pass category. Immediately, it’s abundantly clear that he was seeing a lot more of the ball. He connected with 52% of the long passes, but the volume meant his overall pass accuracy was down at 68%. Instead of highlighting what happened, there’s an opportunity to explore why it happened.
Sample size is useful too when analysing players linked with moves.
Gleison Bremer is reportedly a target for a number of Premier League clubs. When glancing at his numbers for this season, he profiles fairly well. He’s winning 61% of his ground duels and 62% of his aerial battles. He’s heavily involved in Torino’s build-up, averaging 55 passes per 90 and his passing accuracy is 91%.
But, the Brazilian has only racked up 1,200 minutes this season in Serie A. The sample size needs to be larger and when looking back at his 2019/20 numbers, you notice a few things.
Firstly, he was only winning 54% of his aerial duels. When comparing the numbers, you see that he was challenging for more. His increase this season coincides with him averaging two fewer duels in the air per game. Same story with ground duels. He’s winning more this season but attempting fewer.
Combine the two seasons and you get a better understanding of the 23-year-old.
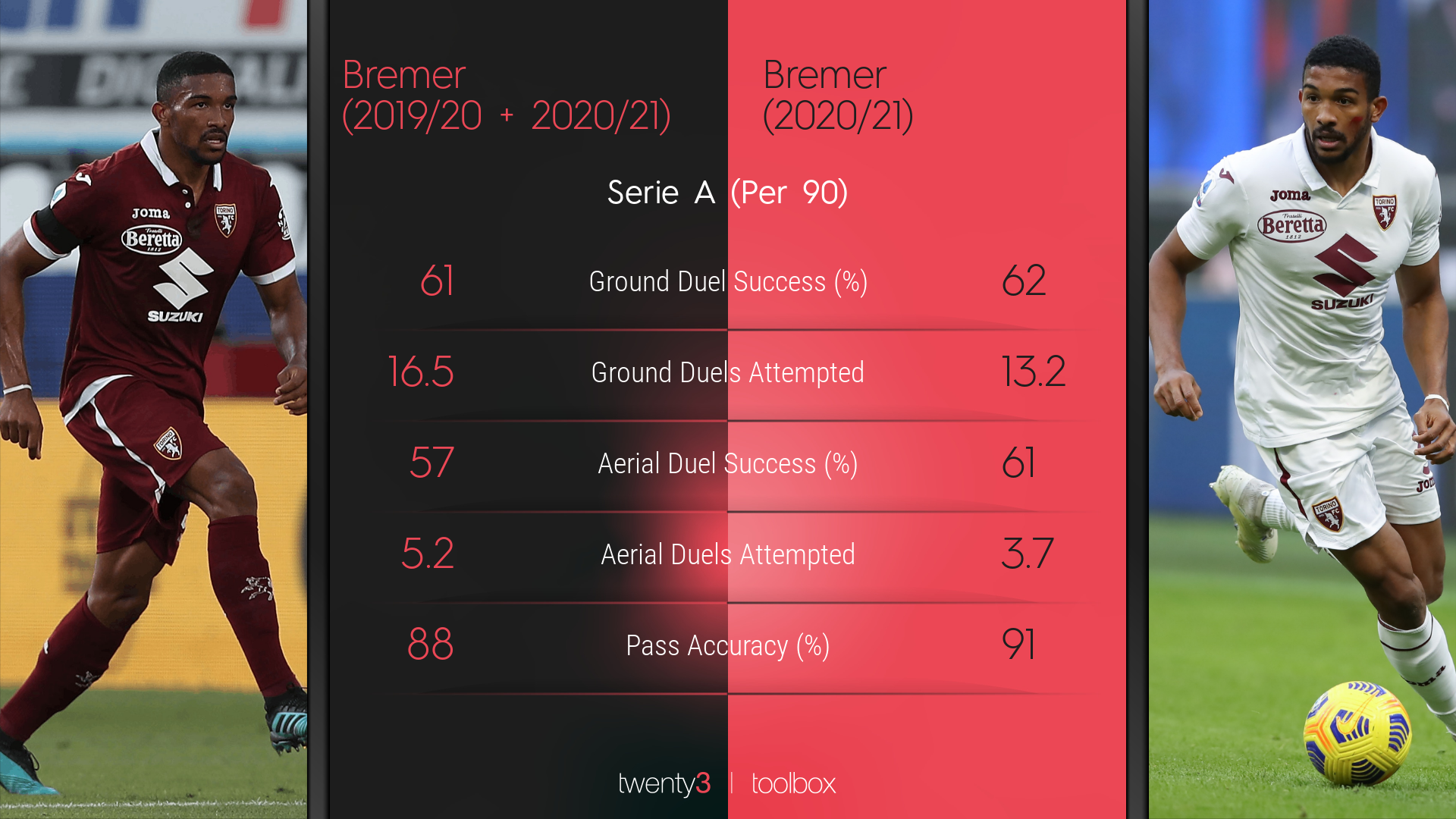
Success in football is built around sustainability. One of the best ways to set yourself up for that is to ensure signings are performing well over a large period of time, and that is why sample size is so important.
All the graphics and visualisations in this article use Wyscout data and were produced in the Twenty3 Toolbox.
If you’d like to learn more about our products or services, and how they might be able to help you, don’t hesitate to get in touch.